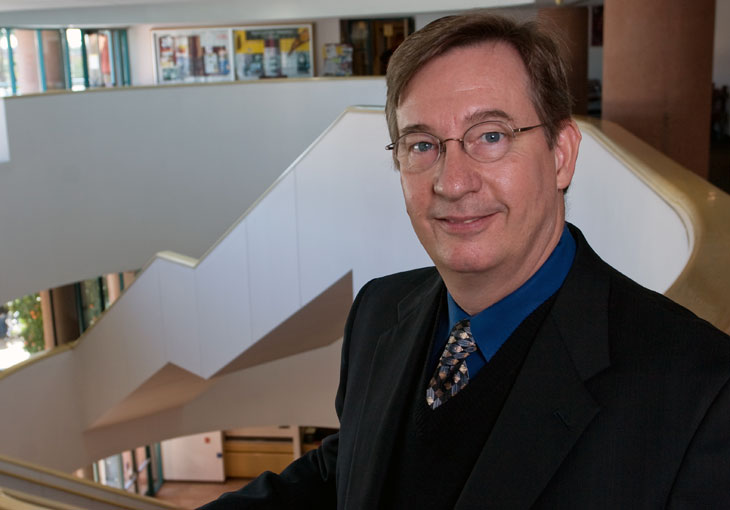
Use prediction markets to raise your marketing model's performance
Which prospects are most likely to buy what you're selling? Who are your good credit risks? What will your customers buy next? Chances are, your company uses predictive models to answer these and other questions, or soon will. A recent study found that 38 percent of companies were using advanced analytics to create predictive models that can forecast consumer behavior, evaluate consumer credit-worthiness, pinpoint marketing targets and more, while 85 percent said they planned to start using such models by 2012. But sooner or later, most of these companies will experience model decay, in which performance lags and it's time to pick a new model. How can managers judge which new "challenger" model will outperform or, at least, replace the reigning "champion?" Information Systems professors Michael Goul and Sule Balkan think one smart bet would be to try a market approach. Recent research conducted by these scholars found that "prediction markets" proved to be an effective approach to selection of predictive models.
Which prospects are most likely to buy what you’re selling? Who are your good credit risks? What will your customers buy next? Chances are, your company uses predictive models to answer these and other questions … or soon will.
In 2009, The Data Warehousing Institute found that 38 percent of companies were using advanced analytics to create predictive models that can forecast consumer behavior, evaluate consumer credit-worthiness, pinpoint marketing targets and more, while 85 percent said they planned to start using such models by 2012. And, sooner or later, most of these companies will experience model decay, in which performance lags and it’s time to pick a new model.
How can managers judge which new “challenger” model will outperform or, at least, replace the reigning “champion?” Information Systems professors Michael Goul and Sule Balkan think one smart bet would be to try a market approach. Recent research conducted by these scholars found that “prediction markets” proved to be an effective approach to selection of predictive models.
Market savvy
What is a prediction market? Also known as information market, it’s a make-believe stock exchange designed to make predictions. The market does this by letting people purchase “stocks” with the aim of predicting events or other parameters, such as top sellers for a corporation’s goods.
“When you invest in a stock market, you’re betting. Whenever there is betting, that’s a prediction market,” notes Balkan. Just as stock-market participants bet that a corporation’s shares will rise, participants in prediction markets are betting about other types of outcomes, such as which candidate will win an election or what movie will be a box-office hit, she explains.
Balkan adds that prediction markets “aggregate information from participants and reveal the wisdom of crowds.” By “wisdom of crowds,” she is referring to a theory posited by author James Surowiecki, who maintains that decisions based on the aggregate wisdom of a group often are better decisions than those made by individuals in the group on their own.
As Goul and Balkan point out in a recent paper, “Prediction markets gained notoriety when a policy-analysis market was created by the Defense Advanced Research Projects Agency to determine if the approach could help to predict future geopolitical events. The market was unique in that it was designed specifically for information aggregation and revelation.”
Today, dozens of prediction markets exist, and many operate over the Internet. The Hollywood Stock Exchange (www.hsx.com), for instance, taps participants’ views on the next blockbuster. The University of Iowa runs the Iowa Electronic Markets to see what the masses think about upcoming election winners, pending monetary policy and other political happenings.
Popular Science magazine ran a prediction market for two years, ending in May 2009. There, the exchange’s 33,000 participants bet on the answers to questions such as “Will total Arctic sea ice drop to 4.25 million square kilometers or less by December 31, 2007?” and “Will the iPhone be hacked to run a third-party program within two months of release?” Both events came true, and market participants had accurately predicted that they would.
Inside corporate walls, prediction markets are being used to guess everything from printer sales to the next must-have gadget or toy slated to dominate the retail world at Christmas, Balkan says.
“Prediction markets often outperform experts and opinion polls,” she adds. In fact, researchers at the University of Iowa compared presidential election forecasts from their own institution’s prediction market to those from national opinion polls going back from 1988 through 2004. They found that 74 percent of the time, the university’s prediction market was closer to the eventual outcomes of the races than the polls were.
As Goul and Balkan explain in their paper, “Markets are made up of diverse people with different levels of information and intelligence. Yet, when you put all those people together and they start buying and selling, they come up with generally intelligent decisions.”
Model behavior
Could companies with decaying predictive models use the market approach to find their next champion model? Given the high cost of misdirected marketing based on decayed models, Goul and Balkan decided to find out.
“Prediction markets operate like stock markets, so when we, as researchers, wondered where we could derive ideas for improving model selection, we decided to derive them from the actual stock market,” Goul says.
To that end, he and Balkan designed an elaborate experiment in which market participants bet on the outcomes of various marketing models based on information displayed in a dashboard. The dashboard served as a market signal, as it reported performance of the assorted marketing models — or stocks — within the made-up exchange.
To see if deep knowledge of predictive modeling made a difference in betting behavior, the researchers used both undergraduate- and graduate-level Information Systems students. And, since a real stock market often sees people buy equities simply because a prosperous friend or relative picked it, the researchers added a social component to their experiment. They gave some people permission to confer with other market participants, and they measured the interaction by giving those participants candies that had to be exchanged to reflect the interaction. Each participant had $1,000 in play money, and those who came closest to the group’s decision earned a $50 reward.
The story behind the betting was that a casino marketing executive named Yolanda Wales needed to evaluate the marketing models produced by her business intelligence team. To do so, she created The Wales Market, in which participants were given a description of each model and background on past performance. After the betting and use of the various models, participants learned the actual and predicted performance from each marketing campaign cycle, and then betting began again.
This experiment proved to Goul and Balkan that such an approach did indeed operate like a prediction market. Different groups — undergrads versus grad student and solitary investors versus social ones — exhibited surprisingly consistent betting behavior, the researchers found.
A market that goes DEEPER
In other work, Goul and Balkan have developed a model-improvement methodology they call DEEPER. The acronym stands for the steps they think companies should be taking when using advanced analytics to guide campaigns. These steps include “designing” campaigns based on predictive models, “embedding” the offer into business processes, “empowering” employees to support the campaign, measuring “performance,” “evaluating” results and “retargeting” future efforts.
“DEEPER is the cycle that you follow to determine whether you need to do some retargeting because the predictive models that you’ve been using are starting to decay to the point where they’re not effective,” Goul explains. “Something has happened that shifted results.”
As part of the DEEPER cycle, Goul and Balkan think that predictive markets could be an effective way to retarget marketing efforts. “Prediction markets apply to model deployment,” Goul says. “That’s the general take-away we came up with from our initial research.”
This research now has Goul and Balkan thinking of new elements to add to their prediction market of predictive models. One element is the social networking phenomenon found in mirrored investing, where market participants choose to mirror the investments made by a master trader of their choice. “Mirrored investing lets individual investors keep up with Wall Street even though they’re outgunned by high-powered trading computers and researchers who buy and sell stocks daily. With mirrored investing, it’s as though you have access to that information in real-time,” Balkan explains.
She and Goul envision this as a possible component for model-selection via prediction markets. Goul also sees mirrored investing coming into play as a means of evaluating automatic investing, such as that which occurs with the same kind of program trading that wrought the “flash crash” of May 2010, when the market plunged 900 points in minutes. “With program trading, there are algorithms watching the market and automatically executing trades,” he explains.
What intrigues Goul about program trading is that it combines human and automaton elements in market activity. According to Goul, a recent study conducted by researchers at the Massachusetts Institute of Technology found that the combination of automatons and people outperformed people alone in prediction markets.
“When you start thinking about a deployment cycle and analytics, some things may move very fast” in the continuous-improvement cycle outlined by the DEEPER methodology, Goul notes. As an example, he points to a campaign being aimed at prospects as they sign up for email information via a website. “In an instance like that, the combination of automatons and people participating in a prediction market may give you a better notion of whether your campaign is on the mark,” he adds.
In the end, however, Goul cautions that a prediction market would be no more than a model-selection tool. “It doesn’t give you the final solution,” he says. “It’s a recommendation. The campaign team would leverage what they can from it.”
Bottom line
- Companies increasingly use predictive models to find the best prospects for marketing efforts, gauge customers’ credit-worthiness, cross-sell, up-sell and more.
- Predictive models decay with time and become less effective, so companies must find new models to replace their champions.
- Since groups often come up with better decisions than individuals alone, two W. P. Carey researchers think one way to pick the best models to replace a champion is through “prediction markets.”
- Prediction markets operate like stock markets, but their purpose is to predict an outcome, such as an election or movie’s popularity.
- Studies show prediction markets often outperform experts and polls. The W.P. Carey School researchers proved that prediction markets could work as a model-selection tool, and they also believe that such markets could become all the more powerful by replicating market trends, such as mirrored investing and program trading.
Latest news
- Lab lessons: Roadcase.com VP shares how ASU's SMB Lab fueled growth and efficiency
The Arizona-based audio/visual equipment case manufacturer gets expert guidance on improving…
- Best installment loans
Loans should be prioritized by their ability to improve human capital, says an ASU finance…
- Why does online shopping make me feel like absolute crap?
Online shopping can cause anxiety and frustration, says a W. P. Carey marketing expert.