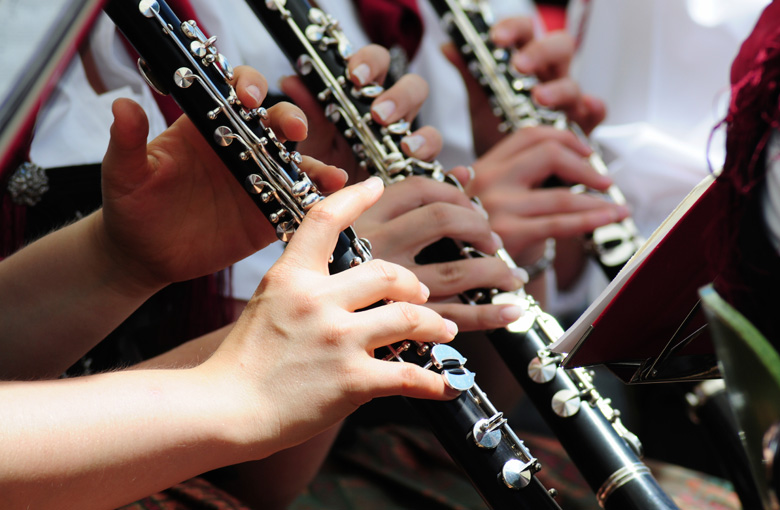
Success in big data requires a symphony of skills
Impeccable data science skills and knowledge, deep business domain expertise and comprehensive information technology prowess: these are three ingredients necessary for success in big data.
By Michael Goul, Chairman, Department of Information Systems
Impeccable data science skills and knowledge, deep business domain expertise and comprehensive information technology prowess: these are three ingredients necessary for success in big data. This trifecta of expertise can be found in the best industry teams assembled to realize big data success. But there’s no preparatory MOOC, no single analytics educational program or any one comprehensive industry or professional society certification that can prepare one to be the ambidextrous utility player. One of my favorite presentations on this very issue was given at an SAS Analytics conference by two experts from the American Express Company: Landon Starr and Pieter van Ispelen.1 The two described an analytics application in CRM where they were experimenting with the value of mining customer conversations — that is, the sequence of events and interactions a company has with a client/customer over a period of time.
The focus is on questions like, “When is the best time to make an offer during a conversation?” Starr and Ispelen claim: “It’s no longer enough to send offers, or think about the effectiveness of an email. We’ve found ourselves in a world where we have the technological means to guide, assess and dynamically adapt our customer conversation.” (Starr and Ispelen, SAS Analytics 2014, pg. 4) Starr and Ispelen use the metaphor of a symphony to describe how data science, business and IT experts need to work together to make advances in the area of data science-driven conversations. They advise business experts to take the lead in understanding segments and IT to provide insights on speed, construction and recommendation execution. The role of data science is to discover through experimentation how to interact as volume flows in, customers respond and conversation track alternatives are vetted. Statistical significance triggers automated adaptations to the conversation.
One of the most interesting applications of the symphony concept is to consider how data science and IT work together. By leveraging historical data trends — to simulate how many customers will filter into each track, what portion of the conversation-event data to capture for maximum dynamic guidance benefit (and at what storage/retrieval cost) and what execution speeds might be necessary for the production setting — the overall estimated cost/benefits of the data science discovery can be gleaned. By working together, IT and data scientists can see a new discovery through to its deployment — and can do their collective best to ensure that there is a return on investment. In the Information Systems Department at the W. P. Carey School of Business, we are starting to learn more about the successes and failures of this orchestration from our students’ experiences. We have business data analytics students working on dual majors in finance, economics, supply chain, etc.
They are combining data science and domain expertise. Along those lines, we also have computer information systems students working towards dual majors in business data analytics — they have their business core and both analytics and IT preparation. And we have MBAs interested in an analytics dual degree, Master of Science in Information Management students with some analytics mixed in, and business analytics students with some IT mixed in. We even have faculty members who teach in two or more of the programs. The symphony is playing at the W. P. Carey School, but we’ll need to keep listening to ferret out the music from the noise. There is never a dull moment in this type of environment! 1Starr, Landon and Pieter van Ispelen, “CRM – Balancing the Speed, Relevance and Frequency of Your Customer Conversation,” SAS Analytics 2014, 10/20/2014.
Latest news
- A new chapter for Sun Devil Athletics
Sun Devil Athletics Director and two-time W. P.
- ASU AI expert recognized for impact in information systems research
Pei-yu Chen was honored for her contributions to the Management Science Journal.
- Data analytics expert receives prestigious award for dedication to information systems community
World-renowned artificial intelligence and data analytics expert Olivia Liu Sheng was honored…