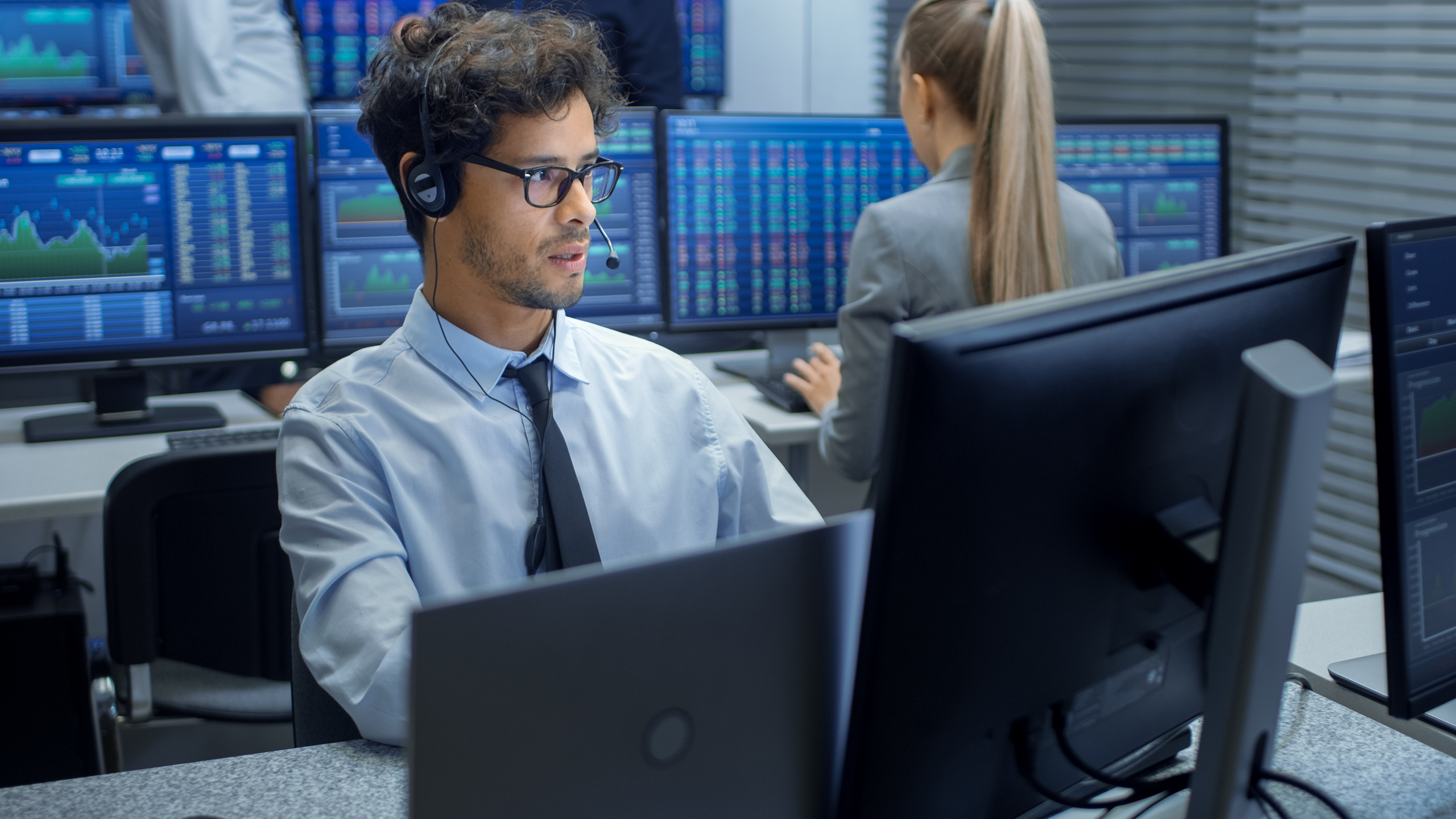
Decoding the mystery of stock return anomalies
Clinical Associate Professor of Finance Geoffrey Smith and his co-author applied a rigorous test to 15 well-known market irregularities. Surprisingly, they found that five held water, bucking established economic theory.
You’ve heard of stock return anomalies — the correlations that predict performance by tying it to a colorful variety of variables. Some claim a single key indicator can tell you how a host of companies will perform. Others forecast market direction by the day of the week or the time of year. Some measures, like the Super Bowl indicator and the hemline indicator, sound downright superstitious.
Yet the claims persist, prompting economists to test them. Not surprisingly, they have concluded that many don’t exist.
But not so fast, says Geoffrey Smith , clinical associate professor of finance at the W. P. Carey School. Though Smith has himself researched and debunked the weekend effect, the holiday effect, and the January effect, he believes the standard test that other researchers use is biased, leading them to dismiss anomalies with insufficient evidence.
For his latest paper, Smith and Russell Robins of Tulane University applied a more rigorous test to 15 well-known anomalies. Surprisingly, they found that five held water, bucking established economic theory. Smith’s point, however, is not to dispute mainstream economics, but to get other academics to use better methods so that a body of reliable evidence can be considered.
Why anomalies matter
Stock return anomalies fly in the face of the efficient markets hypothesis, developed in 1970 by economist Eugene Fama, who won a Nobel prize. It states that markets factor in all available information about a stock to determine its price, making it impossible for investors to gain a consistent edge — with the emphasis on “consistent.”
There may be blips. But true anomalies — unexplained phenomena that consistently lead to profits year in and year out, so that all you have to do is press a button and open your wallet? Not possible, the theory says.
Why? Because investors would pile into these stocks, causing their prices to rise so precipitously that they’d no longer be worth the effort. If long-term anomalies do exist, they would undermine the theory. That’s why economists are studying them.
“Are these things just temporary blips, or are they something permanent? If they’re permanent, it would violate our normal way of thinking,” Smith says.
Debunking the debunkers
Many researchers believe they are disproving anomalies, but the method they use doesn’t do that, according to Smith. “People trying to shoot down anomalies are not doing it the right way,” he argues.
They go wrong by comparing results from the paper that originally demonstrates the anomaly — data that, by definition, supports its existence — to data in other timeframes they select. Some use a period before the anomaly was first published as a comparison. Others use later time, and still, others use a span including some or all of the original study period.
But no matter how you slice it, choosing one timeframe and comparing it to another in which you know there is an anomaly will skew your results, Smith says. To explain, he uses a hospital analogy.
“Suppose you want to test whether hospitals make people healthier, and you look at the health of people who went to the hospital and compare it to the health of people who didn’t go.” The people who went to the hospital were already sick, so it’s not a valid comparison.
The same logic applies to anomaly testing. Just as it’s not fair to compare healthy people to sick people, it’s not fair to compare “anomaly-healthy” results — those that you know demonstrate the anomaly — to results in other selected timeframes.
To demonstrate his point, Smith ran 100,000 Monte Carlo simulations that showed researchers who apply a standard constancy test to comparison timeframes aren’t calculating what they think they’re calculating. Instead, they’re rejecting anomalies too soon, without adequate data to support their conclusions. If they had gone farther back and forward in time, their judgments wouldn’t have held up.
A comprehensive test
To eliminate this problem, Smith uses a different test called Quant/Andrews. For this study, instead of selecting a timeframe to compare to original anomaly results, he went back as far as possible for each of the 15 anomalies he tested — back to 1926 for the earliest — and incorporated all available data about them. His vast trajectory included all of the original study periods as well as years before and after, extending to 2018.
For each anomaly, he compared the first month’s result alone to that of all the other months combined. Then he added months one and two together and compared that result to all the remaining months. Then months one, two, and three … and so on until he had repeatedly interrogated every anomaly under the harshest possible light.
“We tested every possible difference, every possible point, with no bias and using all possible data,” he says. The results showed that five of the 15 anomalies were completely worthless. Of the remaining 10, five consistently had an effect, but not enough for traders to make money on.
The other five, though, held up to his rigorous testing. These anomalies exist, but the study doesn’t explain why, or why investors haven’t piled in and negated them. Part of the reason could be implementation, Smith says. High trading costs or fear of trading indicators others don’t use may have prevented investors from jumping in.
But beyond that, he doesn’t know why these five anomalies persist. “Everyone knows about them and we teach them, but no one can explain them,” he says.
If more researchers adopt Smith’s testing methods, patterns for these and other anomalies may someday emerge, providing insights on what remains a mysterious, intriguing problem.
Five true anomalies
Here are the five stock return anomalies that held up under professor Geoffrey Smith’s scrutiny. If you’re feeling brave, you can try trading them. Smith won’t be joining you, though — his passion is economics, not stock trading. “I just buy the S&P. You beat half the market that way. I’ll take that,” he says.
- Momentum: Buy past winners, sell past losers.
- Accruals: Accruals sum up expected revenue and expenses that haven’t yet hit a company’s books. Buy low-accrual firms and sell high-accrual firms. “Maybe high-accrual firms are less reliable in their financial reporting,” Smith suggests.
- Book-to-market ratio: A company’s book value divided by its market capitalization (stock price times the number of shares). Buy high book-to-market firms and sell low book-to-market firms.” Maybe high book-to-market firms are riskier,” Smith says. In the trading world, greater risk often means greater return.
- Net share issues: The number of shares a firm issues. Buy low-share-issuing firms, sell high-share issuing firms. “Maybe managers issue shares when they know the firm is overvalued,” Smith says.
- Price-to-cash-flow ratio: Stock price divided by operating cash flow per share. Buy low-ratio firms, sell high-ratio firms.
Latest news
- Buyers gain upper hand in Valley housing market
Arizona housing inventory is growing, but it's not yet a true buyer's market, says W. P.
- Wisdom from the supply chain OG
A W. P.
- MACC graduate Kailey Shupe finds immense value in program’s data analytics focus
The Master of Accountancy and Data Analytics (MACC) at W. P.