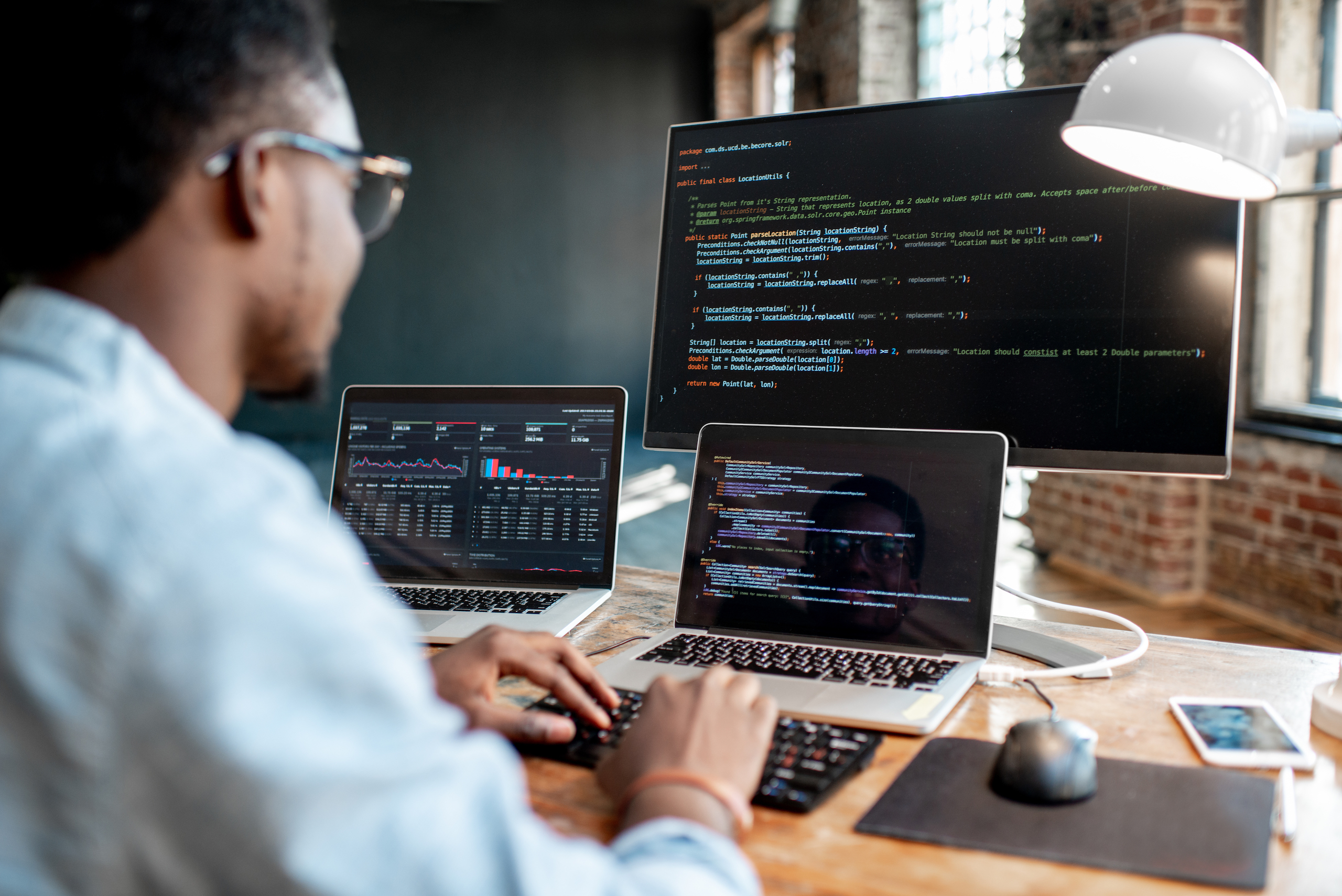
Can technology predict whether a company should take on debt or not?
New research that uses machine-learning algorithms — like the ones that block email spam and detect credit card fraud — answers whether a company should issue stock or bonds to finance their future operations. Ninety to 92% of the time, it predicts what is likely to be the correct debt-equity decision.
Whether to take on debt or not to take on debt — that is the question. It can be a life-or-death decision for a company, especially as the U.S. economy appears to be sliding into a recession.
Now Clinical Professor of Finance Geoffrey Smith has found an extremely accurate way to predict whether firms needing new capital should issue stock or bonds to finance their future operations.
"It's a big decision for a financial manager because it's going to affect the future cash flows of a company. If you make the wrong decision with debt, especially now with the economy possibly heading into a downturn, your firm can go bankrupt," says Smith whose research focuses on data science and machine learning.
His groundbreaking study used machine-learning algorithms — like the ones that block email spam and detect credit card fraud — to predict whether a firm should issue stock or bonds, marking the first time this increasingly common technology has been applied to this question. Ninety to 92% of the time the programs "learned" to predict what is likely to be the correct debt-equity decision.
Following in other firms' footsteps
Smith's finding could have profound results for managers. "If it turns out that firms with characteristics similar to yours decided to issue bonds 90% of the time, and you're planning to issue stock, you might want to think about why you're planning to do something that the algorithm predicts 90% of similarly situated companies would do differently," he says.
He is quick to caution that his findings say nothing about the correctness or incorrectness of an individual company's decision only that the algorithm's results can inform executives what other firms have done. "A CFO can use the results to validate his decision, but it doesn't predict whether his decision is going to work out," he says.
Other non-sophisticated techniques have been used to study the debt-equity decision, but Smith's study is the first to use machine learning to evaluate it.
He speculates that company leaders could apply this method to get a competitive advantage. "If you're curious about what you think a competitor would do, you can feed its data into it. With 90% accuracy, you can know what its choice will likely be," he says.
Foundations of finance
A company's decision whether to seek debt or equity is the foundation of finance, according to Smith, who received his PhD in finance from the University of Georgia in 2006 and teaches MBA students courses in international finance and corporate finance. "It's the reason financial executives exist," he says.
Growing firms typically prefer to issue stock to finance growth opportunities, especially if their stock is rallying, because they will want to take advantage of rising stock prices. On the other hand, firms with tangible assets, such as machinery, land, and buildings, tend to issue debt, because those assets can be collateralized. Plus, if a company has less debt than its competitors, it may want to issue debt because it is not using enough compared to its peers.
Paying the price for high debt
In recent years, however, debt has become a trap for many corporations, even some of the world's best-known. For example, Hertz went bankrupt in 2020 after issuing complex financial instruments for 15 years. As a result, it ended up owing $12,400 for every car worth $10,000 that it owned. Then when the car rental giant could least afford it, its creditors exercised their option to demand their money back and caused the company's collapse.
What's more, many corporations went on a debt binge due to COVID-19. Today, the total debt of U.S. corporations stands at $11 trillion, according to the U.S. Federal Reserve, a staggering figure that is about half the size of the U.S. economy. In a downturn, many of these companies could be punished because their borrowing costs will be raised, according to Smith.
Applying more machine learning in finance
To conduct his study, which was limited to publicly traded companies, he used data from Compustat, a quarterly database published by Standard & Poor's that covers thousands of companies in all industries.
After excluding utilities, financial companies, and non-profits, because of their non-standard operating models, Smith calculated debt as the total of both short- and long-term obligations. He only included companies that had a market-to-book ratio between 0 and 25. For the study, he limited new debt and equity issues to amounts that ranged between 5 % and 250% of a company's total assets. The total number of companies studied was 1,067 debt-issuing companies and 453 that issued equity, all of whom were randomly selected.
Smith hopes his paper will stimulate other research using machine learning on the debt-equity question. "I got 90 to 92% accuracy. I'd like other researchers to take up the challenge and try to get even more accurate results — perhaps 100% accuracy — using other techniques or other data," he says.
Smith's paper, "Predicting the Debt-Equity Decision," appeared in the publication Finance Research Letters in August 2022.
Latest news
- Musical instruments would get more expensive under Trump's tariffs
Increased prices could limit who learns to play an instrument, says an ASU supply chain expert…
- Why wealthy Americans work
An ASU economist's research shows that the affluent don't work for more stuff, but for better…
- ’Big league’ or big illusion? Study calls time on splashy stock market anomalies
In his latest research, an ASU professor invents a stock market anomaly to expose the shaky…