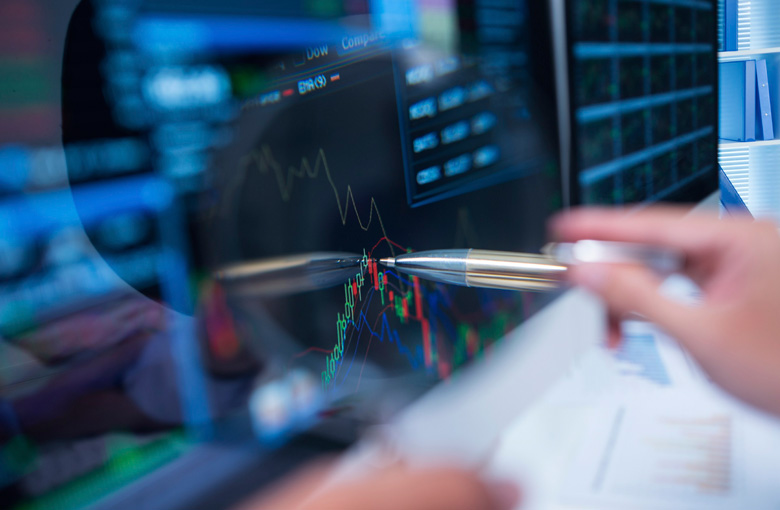
Can your BI live long and prosper? Not without proper governance
For every CIO, Michael Goul has this question: Is your role like the original Star Trek’s Mr. Spock or Scotty? How you answer depends in part on how your company approaches the governance of predictive analytics, data modeling and business intelligence activities.
First published in KnowIT June 29, 2015
For every CIO, Professor of Information SystemsMichael Goulhas this question: Is your role like the original Star Trek’s Mr. Spock or Scotty? According to Goul, how you answer that question depends in part on how your company approaches the governance of predictive analytics, data modeling and business intelligence activities.
There is no right answer – they’re just different CIO leadership roles. He sees it this way: “Scotty CIOs keep the engines running, and they might complain that execs are pushing them too hard. Their job is one of the most important in the company – it’s to deliver cost-effective enterprise computing value. Other CIOs, the Spocks, will be at the helm, helping the captain pilot the ship.”
If CIOs get a governance program in place early to guide their organization’s predictive analytics approaches, Goul says they’re involved in important front and center business innovation issues. “That makes them more like Mr. Spock,” he adds. For some companies, Scotty’s are transitioning to Spocks given the increasingly recognized value of business intelligence and analytics. Goul, who is also the W. P. Carey School's Associate Dean for Research, is spreading this message for the Society of Information Management’s small and elite cadre of CIOs known as the Advanced Practices Council (APC). That group is a forum of senior IT executives who bring transformational solutions to their organizations by commissioning research and sharing cross-country industry perspectives.
For the APC, Goul has lately been engaged in research related to infused analytics and the algorithms stemming from them that inform applications, marketing models and more. Specifically, he’s looking at the governance that might keep these BI tools and models from failing. “Organizations need automation to get speed of processes, but sometimes you need to recognize that analytics decay over time,” he says.
Out of sight, out of mind
Just what are infused analytics? They’re the algorithms and models that companies develop based on data available and a host of assumptions, then embed in a device, program or application, at which point “the infused analytics start running the show,” Goul explains. As an example, Goul points to a tale he heard about a large bank’s approach to issuing credit cards and extending credit limits. Back in the 1970s, someone realized that the same people who carry balances from statement to statement and don’t necessarily pay bills on time were the most lucrative customers for the bank.
After all, they regularly paid late fees and interest. But, all the predictive lending models the bank was running were pitching these money-makers to the curb. As a result, one intrepid data analyst went to IT, hoping to get the code changed to embrace the over-extended rather than rejecting them. When IT managers declined the project, this same data whiz learned to write the code himself, and he inserted it into models that governed lending practices for some three decades. “During the recession, the bank got bit because the environment changed,” Goul says. That’s because the context and behaviors governing the lending philosophy in the infused analytic had changed.
This isn’t the only instance Goul offers of analytics gone bad. He points to flash crashes from algorithmic stock trading that can bring a market down – and up – in seconds. Another example is found in the real-estate-based collateralized debt obligations – the CDOs – that evolved into investment derivatives. Warren Buffet calls them “financial weapons of mass destruction.” Even the leading financial research firms missed seeing how risky these were before they contributed to the great recession. Goul once had the awkward experience of bringing this up at a small-venue lecture attended by one such financial firm’s CIO. He says other audience members shifted uncomfortably in their seats, but the CIO was willing to face the issues at hand.
According to Goul, the CIO admitted that his organization’s models had decayed, and there was no one watching to assess whether these derivatives were outside the bounds of the assumption made when the models were built. “A lot of the time we can’t easily figure out what’s gone awry,” Goul says. “If an analytic has been infused for a long time, the people who built the model may not even be around anymore.”
From-the-trenches insights
To remedy this situation, Goul is collecting best practices from some of the largest, most innovative users of analytics around and beginning to codify them into a set of governance guidelines. Among the best practices Goul has gathered, you’ll find a three-tiered infrastructure approach at Dell. Data scientists at this computer company can do anything they want, provided they’re playing in the sandbox tier of the enterprise environment. IT generally keeps its hands off systems tier, leaving the data scientist to explore at will.
Once a model gets generated, Dell tests it in a semi-production tier of the environment, an area for enhancing maturity and standardizing the new metrics or analytics. In this tier of the environment, IT monitors performance and recommends optimizations. “They use this area for testing and hardening off their models,” Goul says. “When something is proven to work, they’ll put it into the production tier, which is owned and governed by the IT department.” Turkcell, a Turkish mobile service provider, showed Goul another smart governance approach. Faced with an abundance of ineffective marketing campaigns, that company launched a model-management program. “They identified 50 of their critical models and set up performance measurements and rules around them, then built dashboards to watch for model decay,” Goul notes. American Express orchestrates IT and stakeholders from functional areas to ensure that demands on IT serve the company as a whole. The example Goul gives on this approach comes from a marketing department effort to gauge when offers should be made to customers for optimal response rates.
To do that, the marketing team experimented with the “window size” of online customer conversations. That is, should the company wait for you to have five online interactions before offering a new product or credit-line extension? Should it wait for 10 online conversations? Once marketers knew what window size worked best, they went to IT and said, “we need to store all these conversations for all these millions of customers we have.” Meanwhile, the company also looked at how much data storage this effort would require — and its cost — balanced against how much sales benefit and profit the marketers would deliver. “They evaluated whether this marketing approach was cost-effective given the IT costs involved,” Goul notes. “They called it a symphony of business and IT,” because the tech team and functional units must work in harmony. “When I tell this story to CIOs, it resonates,” he adds. “The data scientists are saying, ‘OK, if we implement my algorithm, here is the benefit.’ But the data scientists don’t know the cost of the infrastructure. IT and business areas have to work together.”
Using these and other case studies, Goul is compiling guidelines for decisions CIOs and other IT leaders must make to ensure predictive analytics and BI activities operate under governance rules that will maintain continuing viability of the models. His research is currently slotting decision making into several categories. Resource management is one. Will you have a hands-off approach, where only IT gets to decide governance issues and do the analytics work within IT-run systems? Or will you divide up the resources as Dell did, into a production environment run by IT, a development sandbox for data analysts and a mid-ground environment for mode testing? Model management is another category. Will you have centralized model management like Turkcell? Or will each functional area manage its models? Process management is yet another consideration. At State Farm, for instance, a centralized team handles the testing of new websites and the algorithms that help them do things like deliver online quotes to prospective customers. And, of course, CIOs who want to fine tune their governance approaches will also want to look at organizational structure.
Will your governance and data analytics talent be centralized or federated among all the various functional areas that use these analytics? “Analytics are moving into so many domains of business, people aren’t sure where to put the data scientists. Does analytics belong to IT or accounting? Where should it be?” Goul asks. Getting a handle on these issues is critical, he adds, because many industry watchers predict that chief marketing officers may wind up with more IT budget than CIOs. What those CMOs probably won’t have is a good grasp of what it takes to mitigate risk to IT systems. That will fall into your lap, Mr. Spock. Or are you called Scotty?
Latest news
- Buyers gain upper hand in Valley housing market
Arizona housing inventory is growing, but it's not yet a true buyer's market, says W. P.
- Wisdom from the supply chain OG
A W. P.
- MACC graduate Kailey Shupe finds immense value in program’s data analytics focus
The Master of Accountancy and Data Analytics (MACC) at W. P.