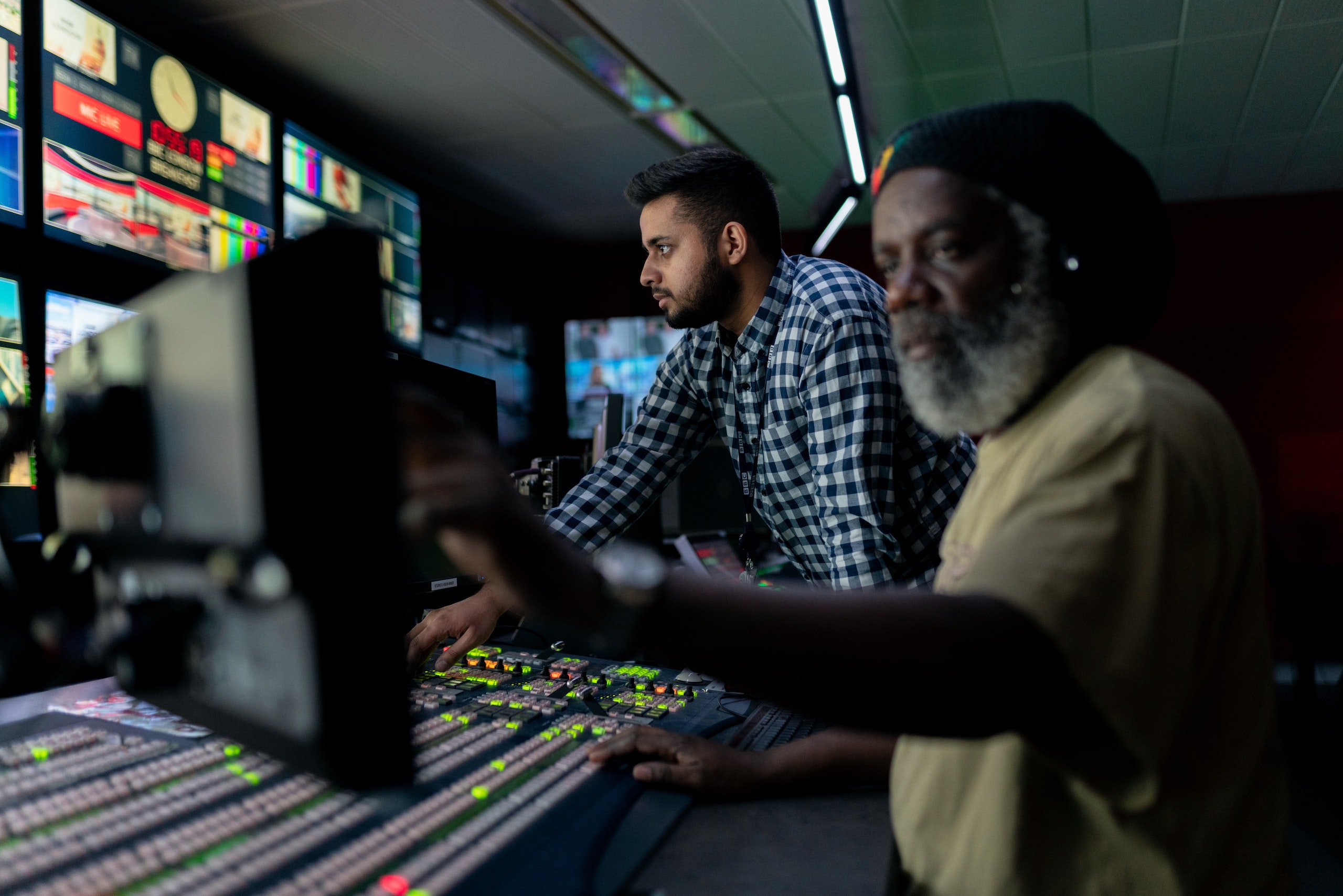
When the government uses AI: Algorithms, differences, and trade-offs
To get a better grasp of AI pitfalls in the workplace, Clinical Professor of Accountancy Gregory Dawson along with fellow researchers studied artificial intelligence systems in the public, private, and non-profit sectors.
Artificial intelligence (AI) isn’t anything new — it’s been around since the late 1950s — but advances in technology have made it commonplace to replace humans with cognitive computing systems (CCS) to get the job done.
Have you ever asked Alexa or Siri to help complete daily chores and get random information? Has a virtual assistant helped you understand the extra charges on your cellphone bill? Maybe you’ve even interacted with Julie over at Amtrak to book you a train ticket? That’s all AI at work.
In addition to chatbots to help customers navigate websites, AI’s application includes predictive analytic systems used for fraud detection, augmented decision-support systems for knowledge workers, as well as fully autonomous systems in transportation, defense, and health care.
For example, the U.S. government uses an AI-based chatbot to interview potential refugees, an application that allows them to answer a series of questions to determine required entrance forms and assess if their status is protected.
AI failures
Yet for all its advanced capabilities and somewhat mythic reputation, AI has faced some real-world issues when it comes to being a smart, safe, and efficient business tool. Police departments have used a popular facial recognition app that is supposed to detect known criminals yet has returned a high number of false-positives and falsely matched 28 members of Congress with mugshots of unrelated individuals.
The city of Chicago also implemented an AI system to help identify people most likely to be involved in a shooting, an algorithm designed to stop those individuals from buying firearms. The problem was that in this case, AI targeted innocent citizens rather than the high-risk offenders it intended to capture with the cognitive automation.
To get a better grasp of AI pitfalls in the workplace, Clinical Professor of Accountancy Gregory Dawson along with fellow researchers Kevin Desouza at the QUT Business School, Queensland University of Technology and Daniel Chenok at the IBM Center for The Business of Government, studied systems in the public, private, and non-profit sectors for the past six years.
What Dawson and his research colleagues discovered is the use of AI in the public or government sector presents some unique challenges — insights that provide a framework for all organizations to create a strategic AI system. Their observations are outlined in the paper “Designing, Developing, and Deploying Artificial Intelligence Systems: Lessons from and for the Public Sector,” which was published in the Business Horizons journal.
Just like Mike
By default, the public and private sectors operate quite differently when doing business, according to Dawson.
There’s a belief that the public sector needs to act more like the private sector and whatever practices are being used can be transferred one to one. But that’s not the case with how AI systems are developed between the public and private sector. You can’t tell it to behave like Google.
– Gregory Dawson, Clinical Professor of Accountancy
“The average company has a unified theme of profit maximization and making money for stockholders," Dawson continues. "That’s not the way it works in the public sector where there’s a cacophony of voices and divergent opinions with many different stakeholders who value different things differently.
"With COVID-19, for example, an epidemiologist suggested we close everything down, while economists said we’d ruin the economy if we did. The Republicans are saying one thing, the Democrats are saying another. And we have companies like Moderna and Pfizer who went to their labs, closed the doors, did research, and said here’s a drug. Compare that to the FDA. Everything it does is highly scrutinized. The FDA can’t just close the door and not be transparent."
For any AI system, the potential for bias is high, where it can’t distinguish between good and bad input. “As part of a larger series of CCS, cognition is what sets AI apart. The system itself learns. Anyone who has sent kids to preschool knows sometimes they learn good things, sometimes they learn bad things,” he says. “That’s the real risk of AI. There’s a stream of stuff coming at you. Some data is based on pretty solid facts. Some are not.”
Such was the case for the city of Chicago, which tried to predict who was going to commit crimes and failed miserably with its automated cognition tool. “The system identified African-American men between age18 and 24 — racial profiling at it’s very best. We just can’t do that,” says Dawson, a former partner in a Big 4 public accounting firm who also holds a PhD in information systems with a focus on the public sector.
Intelligent trade-offs
For those in the public sector who are tasked with building AI systems, the solution is that implied trade-offs have to be made between saving money, public good, security, safety, and scrutiny — complexities private industry normally isn’t up against, according to Dawson. “We expect the government to be transparent in their actions. You have to show process, data, and calculations so there isn’t implicit or inherent bias in the algorithms. If done correctly, you can remove all the biases.”
But the rub is that the government doesn’t have the people and skills to develop systems like this. “With every system, you have to think about people, process, and technology. The government doesn’t typically have professionals to develop AI systems and the existing technology is incredibly dated. It’s like trying to put a jet engine into a 1975 Ford Pinto,” he adds.
Plus, the government is trying to do something it’s never done before with AI. “We have all this data and the government has captured only a tiny segment of it and doesn’t have a sense of how good the data is,” Dawson says. “Our approach in this paper is to explain how the government is different from non-government in a couple of ways. We know AI is the next big thing and has the capability to be transformative. What we are afraid of is the government is going to be pushed by zealous politicians to just do it. But they need to accomplish a few things before they can get to that level.”
How does the government achieve the huge benefits and value that AI offers without blindly rushing to take advantage of its power? If it wants to get from where it is to where it wants to go, Dawson and his co-researchers suggest the public sector, like the private sector, should consider four factors to evaluate its AI needs: data, technology, organizational, and environmental.
To pull it off, the government must make certain it has enough clean, quality data as well as the necessary level of technology and skilled in-house staff to design and implement an effective AI system, according to Dawson. Plus, it has to create an organizational process for implanting AI, share experiences, deal with transparency, and be confident its AI system, when subject to scrutiny, will stand up.
“AI is as transformational to the government as the internet was to e-commerce, and 100 times more important. It’s the future,” Dawson says “Based on examining this, we’ve learned some good lessons and gotten a better idea of what to do.”
Latest news
- Musical instruments would get more expensive under Trump's tariffs
Increased prices could limit who learns to play an instrument, says an ASU supply chain expert…
- Why wealthy Americans work
An ASU economist's research shows that the affluent don't work for more stuff, but for better…
- ’Big league’ or big illusion? Study calls time on splashy stock market anomalies
In his latest research, an ASU professor invents a stock market anomaly to expose the shaky…