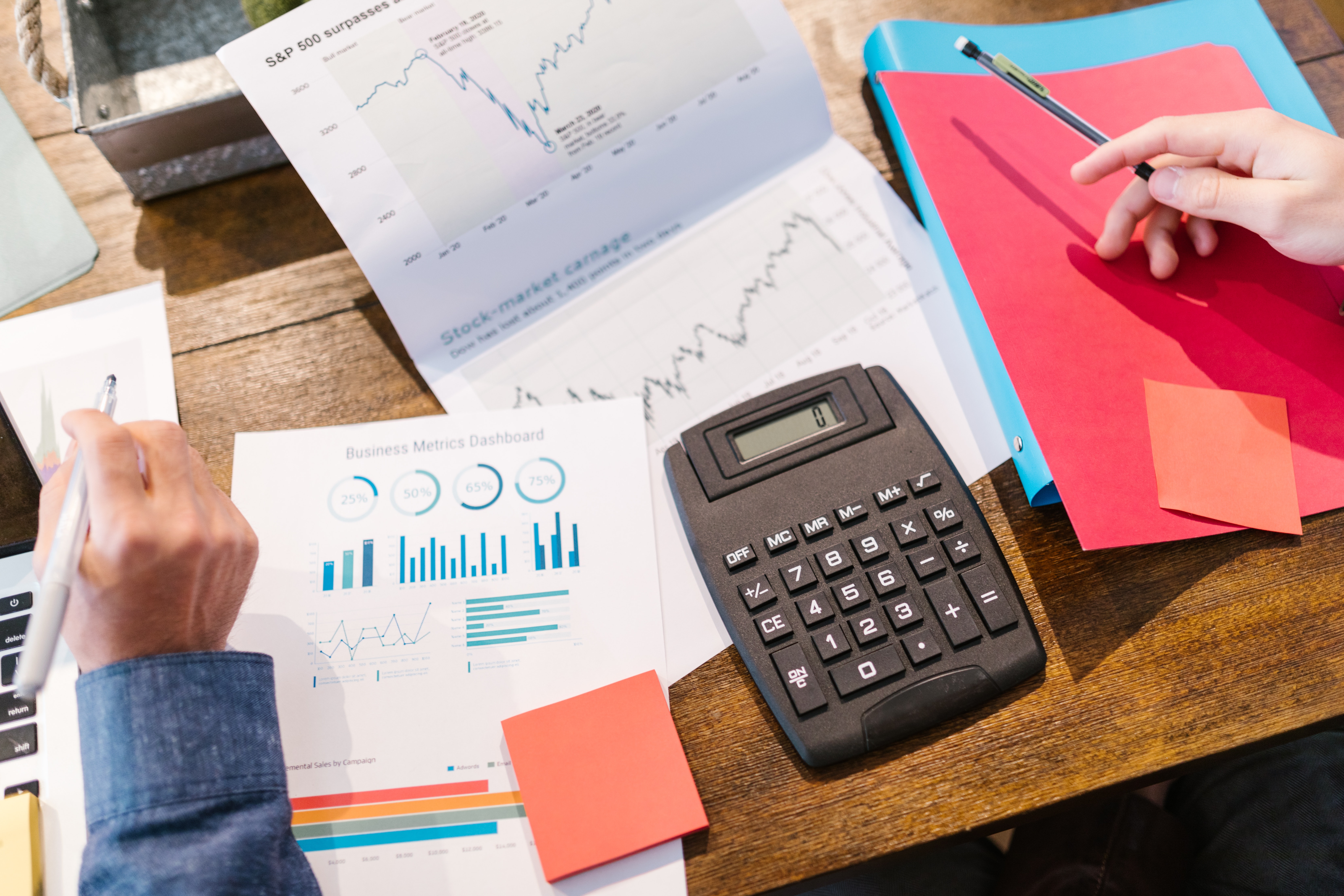
Finding the funding sweet spot
Crowdfunding is a booming business model with more than 6 million campaigns worth an average of $7,300 each in 2022. But how do you know if your Kickstarter project will be a non-starter or if your Indiegogo idea will go far?
Trying to understand why certain crowdfunding ideas are successful inspired Jihoon Hong, assistant professor of marketing, and fellow researchers, Yanhao "Max" Wei and Gerard Tellis, both from USC Marshall School of Business, to write a paper called "Machine Learning for Creativity: Using Similarity Networks to Design Better Crowdfunding Projects." The paper was published in the Journal of Marketing.
"We found it difficult to make a successful crowdfunding project," Hong explains. "The success rate is about 35%. When we look at the highly successful groups, those getting more than $10,000 for their project, it's much rarer." Those results led the researchers to explore how they could equip crowdfunding platforms with metrics to help creators plan more successful projects.
Specifically, the team was interested in finding out whether the similarity network between crowdfunding projects could help predict success based on combinatorial creativity, a theory that suggests every new idea is a recombination of existing ideas.
A recipe for success
Evaluating creative ideas is a bit like dissecting a recipe, Hong says. "There are no new ingredients in this world, but what makes a difference is how you combine the different ingredients to make a unique recipe. The same holds in terms of coming up with a new crowdfunding project."
Hong says it helps to think of each idea as an ingredient that can be used to predict how successful the recipe will be based on the theory of combinatorial creativity. When predicting funding outcomes for a new crowdfunding project, he says the key determinant is what types of projects donors have supported in the past and what components of those ideas, or "ingredients," they were most drawn to. The historical funding outcomes of Kickstarter projects provide a robust database from which to work, allowing for comparisons based on the project concept, how much money it raised, and in what period.
To understand how all those elements are connected, Hong and his fellow researchers needed a way to operationalize the combinatorial creativity concept into a very specific context. They applied machine learning techniques and natural language processing to the descriptive texts of crowdfunding projects. That allowed them to objectively measure the similarity between each combination of ideas and construct a similarity network of ideas. Using this network, they set out to investigate four ideas:
- If the success of similar prior projects can help predict a new project's funding performance
- Whether a project's novelty is rewarded or penalized
- What a project's optimal funding goal is
- Whether a project's unconventional use of prior ideas hurts or benefits funding performance
They discovered that the success of similar projects is a significant predictor for the success of a new project. "We found that, when we compare a new project to the prior similar ideas on the platform, if more and more prior similar projects succeeded, then this new idea is also likely to succeed," he says. "In terms of the number of ingredients, if the idea has too few or too many ingredients, then it's not likely to be successful."
Previous literature on creativity argued that there is a tension between novelty and similarity, meaning an idea should not be too novel or common to succeed. That tenet held in their research, finding that there's an inverted U-shaped relationship between novelty and funding performance — being either too novel or not novel enough hurts the project's chance of success.
The paper also looked at the level of fundraising a project creator set as a goal. "Goal setting is one of the most important aspects of crowdfunding," Hong says. "If a new project sets its funding goal very close to what similar projects raised, the new project will be more likely to succeed." Goals set too high or too low compared to similar projects performed poorly.
Finally, they investigated how important the relationship between and among these prior similar projects is. Like with the novelty level, they found an inverted U-shaped relationship between the extent of atypical imitation in the network of prior similar ideas and the funding performance of a new project. That means it takes just the right balance of atypicality in an idea or project to make it more likely to succeed.
Everyday applications
So, what kind of change can be kickstarted with this measurement technique?
"Let's say I'm the manager of a crowdfunding website," Hong offers. "When I get the text description of your idea, I can compare it to previous postings. Then I can know which ideas are very close to your idea and measure the similarity among all the ideas."
Using their model, platform managers can help project creators find the sweet spot for their ideas by understanding the level of success of prior similar projects and the number of projects that are like their idea. They can also compare the goal funding level of a new project and the amounts raised by prior similar projects and see how many atypical or independent projects within that network were successful. By extracting these four dimensions and comparing your project to the optimal level of success for similar ideas, they might recommend some projects to look at. For example, if your ideas are far from optimal, they can recommend some methods for crafting a project description that sits at the ideal spot in that U-shaped curve.
Hong says that although their research focuses on crowdfunding, their approach can be applied to other text-based creative settings, such as new product concepts, mobile apps, and blogs. For example, with blogs, the machine learning methodology could be used to ascertain whether novel or familiar content appeals more to consumers or even what length of blog they want to read.
Latest news
- Balancing boardrooms and books
How DBA student Jean-Paul Destarac is applying research to real-world supply chain challenges —…
- Second annual Student Economics Association Banquet bridges generations
W. P.
- Recent pause on US and China tariffs
An ASU supply chain expert weighs in on how tariffs are impacting the supply chain and when…