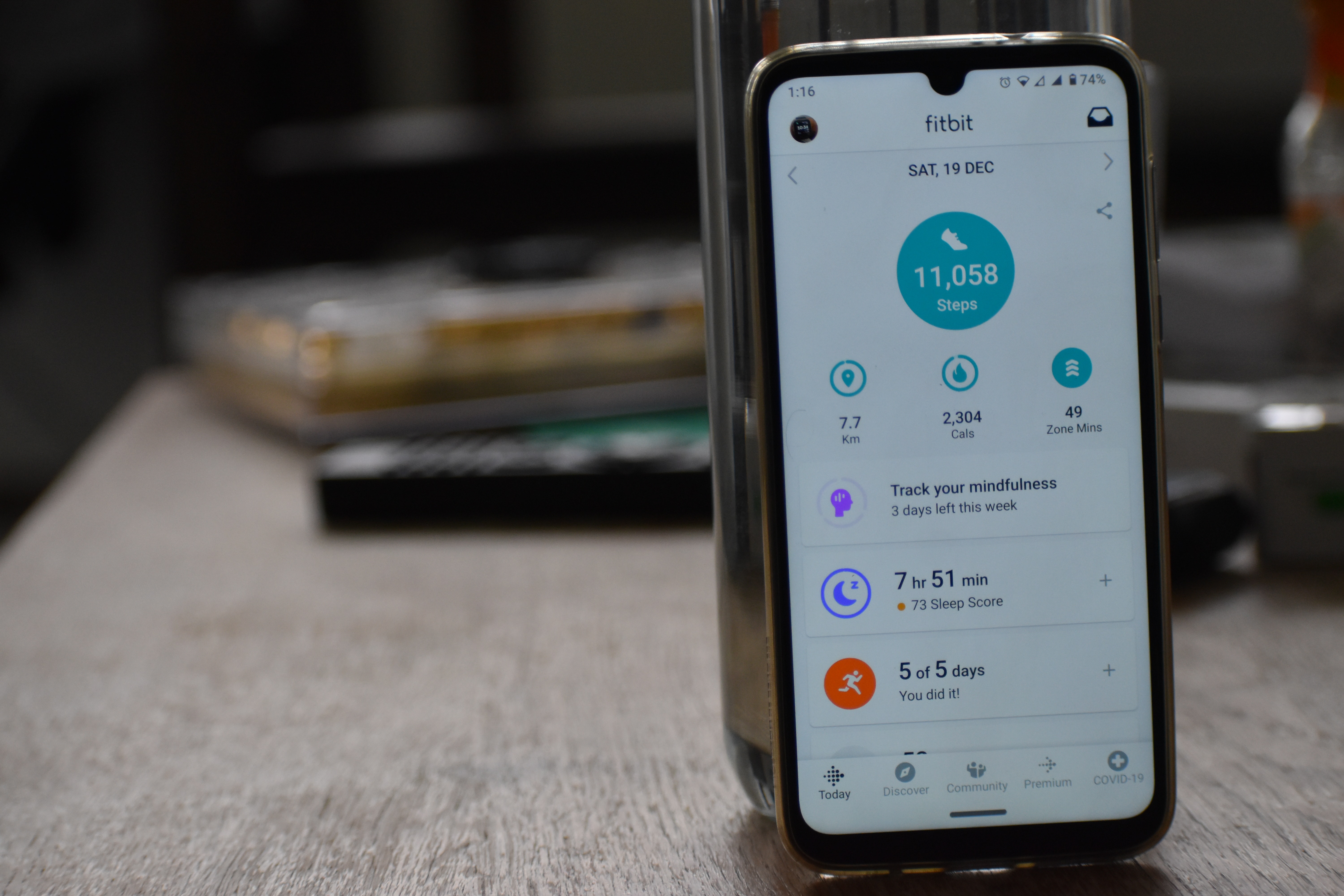
Health care apps could be more effective with an online learning approach
ASU expert says user engagement is crucial to changed behaviors and improved health when using health care apps.
An estimated two-thirds of Americans are overweight or obese. Maybe that’s why 40% of people surveyed by the business intelligence firm Morning Consult last January said they use health care apps, such as calorie and fitness trackers.
These apps can be very effective tools. One study of 36,000 Noom users found that 78% lost weight. “User engagement is essential for health care platforms to help people improve long-term health,” says Tongxin Zhou, assistant professor of information systems.
Zhou has found a way to increase user engagement by enhancing the recommendation system that offers user action ideas. Better engagement improves outcomes in apps supporting weight loss and chronic disease management.
Spoiled for choice
Anyone who’s ever found a great new movie to watch on Netflix knows recommendation systems can be a great helper, but they have drawbacks, too. One is information overload, which can happen when faced with something as potentially complicated as a health care app.
“Nowadays, we talk a lot about preventing illnesses before they become more severe,” Zhou notes. “Prevention is done through behavior or lifestyle changes or interventions, and health care platforms provide these interventions.”
Users may get instructions on calories in foods, what to eat, how much to eat, as well as recipes and exercises to try, she explains. “The platforms provide many options, and users must select which one to take — either a diet or exercise intervention or users can use multiple interventions. The decision is up to the user.”
She adds that when people face too many options, they could wind up overwhelmed. “You don’t know which one to choose. It’s called decision-making paralysis, and it will be bad because you’ll probably stop participating in these intervention programs.”
Despite the risk of overload, studies have found that well-diversified interventions — offering plenty of options to users — help sustain user attention and reduce dropout rates. This motivated Zhou and her colleagues to create a personalized health care recommendation system to deliver tailored suggestions to users and maintain high user engagement.
Zhou and her team moved beyond traditional batch-learning-based recommendation systems to accomplish this. They leveraged a specialized machine-learning algorithm that adapts to real-time data to detect dynamic changes in user preferences. This real-time, online machine-learning approach also facilitates occasional deviation from known user preferences, which allows users to discover other relevant options for their health management regimen.
Stuck in the past
Batch-learning-based recommendation systems use historical data such as prior purchase behavior or reviews to infer the best options the user will like. Zhou says this often works well with things like music, books, or video preferences because people enjoy the same or similar types of movies, music, and shows year after year.
Health care is different; she points out. “If you think about chronic conditions, you’ll see they evolve,” she says. “Take diabetes. Your blood sugar may change based on your prior behavior, so we need to consider behavior to infer preferences in the current moment accurately.”
Suppose a diabetic is trying to lose weight with an app. A batch system may look at the preferences shown in weeks one and two of that effort. Come week three, it will build on those past preferences and may miss changes in the user’s health, weight, or other factors that will impact choices.
Because Zhou et al. used a real-time, online machine-learning algorithm called a “bandit” approach, they’re looking at more data and can offer more relevant recommendations.
“In health care, you need to think about many types of information to describe an individual’s status more perfectly,” says Zhou. “You need to incorporate static information — things like age, gender, and initial weight — and also behavior and health sequences, like your prior health histories, prior intervention participation histories, other health management experiences, and so forth.”
Sequential data — data that reflects inputs in time — also have correlations to be considered in a more precise recommendation system. “What you did in the past affects your behavior in the future,” Zhou says. That’s called a within-sequence correlation. An example of a between-sequence correlation is that health history will affect intervention participation.
For instance, someone with bone-on-bone arthritis of the knee isn’t going to take up running, no matter how many times a recommendation system offers it as an option. Likewise, someone with weight issues may also deal with sleep issues, high blood sugar or cholesterol, and other health concerns simultaneously. Consequently, Zhou and her team tried to incorporate this comprehensive information into its recommendation system and offer the most relevant options to address various needs.
Toward this end, the team used embeddings, which represent data so that points of similarity cluster together. “The embeddings are special codes that the computer creates to understand the unique qualities of users and the interventions you are recommending. So, it can find similarities between things even if they’re not the same. It’s a bit like how humans might see patterns and connections between different items. These embeddings help the computer make better recommendations, finding things that fit each person’s preferences, even if they’re not always obvious matches,” she explains.
The benefit? Zhou’s approach allows the recommendation system to expand beyond past choices and discover new ones.
Zhou and the team tested their approach against batch-based recommendation systems through simulation, and the bandit system was a clear winner. Looking at user improvement — the percentage of users who received more preferred options from the bandit recommendation system versus batch-learning-based ones — Zhou’s approach earned a 97% rate of user improvement. The batch models delivered 20% to 80% of this measurement because user improvement means they’re more likely to persist in their app engagement.
Since Zhou’s research simulated users’ weight-loss outcomes, lost pounds were evaluated, too. Batch-learning-based systems produced in-period weight loss in 60% of users. Zhou’s approach achieved in-period weight loss for nearly 74% of the individuals represented in the model.
Zhou noted that user engagement is crucial for health care apps like weight-management tools. “People must engage in this process to change behavior,” she says. Her model delivered the engagement apps required.
Such engagement improves the health of the users and platforms alike. “Healthcare platforms need to have a substantial amount of individuals who keep participating to attract resources, share knowledge, and make things work,” Zhou explains. “User engagement means better platform sustainability.”
Latest news
- Beer sales research brews up lessons for businesses
New study shows how full-strength beer laws boosted grocery sales through smart product pairings…
- Darknet secrets: Who shares, who hoards, and why it matters
New research reveals how hacker status influences knowledge-sharing — and what it means for…
- MBA grad, entrepreneur Daniel Valdez founded company to help underrepresented groups reach more business leadership roles
As a former member of the U.S.