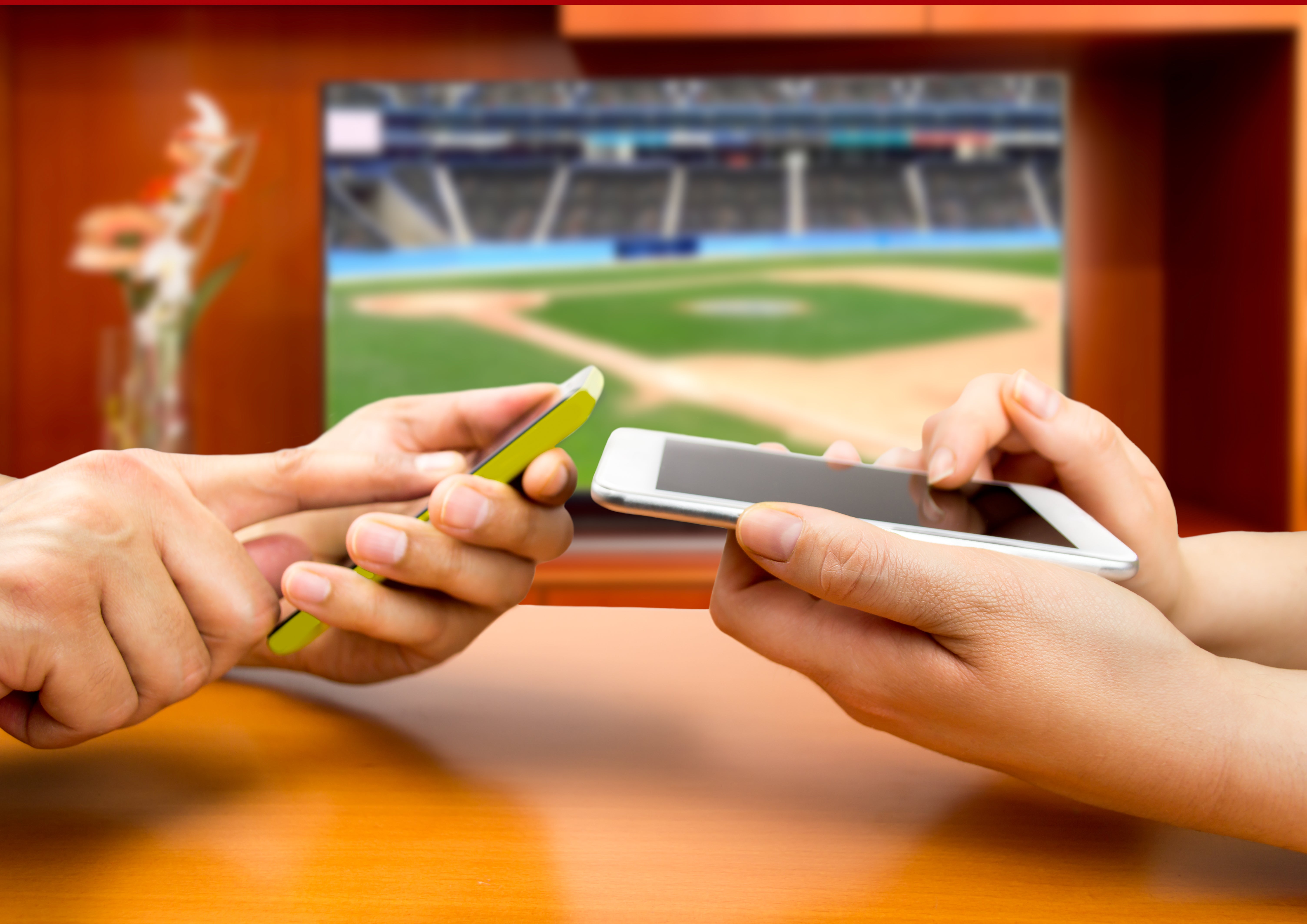
’Big league’ or big illusion? Study calls time on splashy stock market anomalies
In his latest research, an ASU professor invents a stock market anomaly to expose the shaky ground behind quirky Wall Street theories.
Lunar phases, sunspots, music sentiment, religious holidays, and even air pollution — are all examples of stock market patterns that researchers claim can predict returns. But a new working paper from Geoffrey Smith, a clinical professor of finance, set to be published in the journal Critical Finance Review, argues that many of these findings are little more than statistical illusions.
His critique targets a growing trend in financial research: The search for eye-catching patterns that, while headline-friendly, may not hold up under closer scrutiny.
To make his point, Smith does something unusual. He invents a "stock-market anomaly" — a pattern in stock prices that seems to defy normal market logic and appears to offer unusually high investment returns.
He calls it the Big League Effect — the apparent predictive power of the win-loss records of New York’s baseball teams, the Yankees and Mets, over future stock returns. According to his results, months following a string of wins by either team are associated with higher returns on various trading strategies.
A trend worth questioning
But Smith is skeptical of the growing number of research papers linking stock performance to quirky events like sports outcomes or the weather. "Are you really going to invest in the stock market because the Yankees won last night?" he says. "To me, that's silly."
The issue, Smith explains, is that regular statistical methods — like the commonly used T-test — assume the data is picked randomly. But when researchers choose what to test after looking at the results, it increases the chances of finding something that looks important, even if it's just random noise. This can make a weak pattern seem much stronger than it is.
That's why the Big League Effect appears significant under the T-test — just like many other studies that get published, even when the patterns they find aren't actually "real."
And the results do look impressive at first: Buying stocks using certain strategies made about 1.5% to 1.8% more money in months after the Yankees had more wins than losses, according to Smith's study. These strategies included momentum (buying stocks that have been rising) and residual variance (focusing on unpredictable stocks).
For the Mets, the results were even stronger, with some trading strategies earning up to 3.1% higher returns — especially those targeting companies with strong profits or fewer new shares, since issuing new equity can reduce the value of the ones already held.
Standard tests suggest these results are unlikely to be due to chance — but Smith argues that's exactly the problem.
"If the Yankees hadn't worked, I would've tried the Red Sox or the Phillies," Smith says, citing other baseball teams. "And if those didn't work, maybe rainbows, or shark attacks. The point is, that you already know the stock returns before testing. You just keep reshuffling until something sticks."
When patterns fall apart
To test his idea, Smith used 600 months of stock market data from January 1974 to December 2023, taken from Dartmouth professor Kenneth French’s well-known online data library, which tracks the performance of popular trading strategies.
He matched this with win-loss records for the Yankees and Mets from Retrosheet, a database of historical baseball games, and looked at 15 common trading patterns to see if any line up with the teams' performance.
To check if the Big League Effect was real or just a fluke, Smith built a model to estimate how likely each month was to be a "winning" one, based on stock returns. He then randomly reshuffled the data an astonishing 100,000 times and ran tests on each version to see what kind of results could happen just by chance.
From this, Smith came up with tougher rules for deciding if a result means something. Normally, researchers use a number called "1.96" — from a tool called a T-test — as the cutoff to say there's a 5% chance the result happened by luck. But Smith showed that when people test lots of different ideas, that bar is too low.
Raising the bar for what’s real
Based on his simulations, the cutoff should be much higher — closer to four or five — to be truly convincing. And at those levels, the chance of getting a result just by random luck is extremely small: A score of four means about a 0.006% chance, or one in 15,000.
When Smith re-tested the Big League Effect using these stricter rules, it didn’t hold up. What first looked like a strong result turned out to be something that could easily happen by chance — and in fact, none of the 15 well-known stock market strategies he tested still looked real after the correction.
"People are testing for significance using tools designed for random samples. But that's not what they’re doing," Smith says. "I think the best insight here is that I found a test to disprove these things."
His broader critique is aimed at the financial research community itself. While most studies are well-intentioned, Smith argues that the pressure to publish "splashy effects" pushes researchers to highlight patterns that don't hold up.
"You can always find a correlation between stock returns and some odd variable if you dig around enough," he says. "But correlation is not causation."
He points to a growing list of studies that link market performance to things like lunar phases, sunspots, music sentiment, religious holidays, and even air pollution. He doesn't suggest these findings are fraudulent — but believes many are likely the result of data mining, not real market signals.
For investors, the implications are clear — if unflattering. In Smith's view, the market is broadly efficient, meaning prices already reflect the most available information, so it's difficult to consistently beat the market by finding hidden patterns.
Trading strategies based on anomalies that appear once and never repeat, he argues, are unlikely to deliver lasting results. "Just because something shows up in one dataset doesn’t mean it'll show up the next month," he says. "It will probably not be a profitable strategy."
Ultimately, his study offers a framework to separate real signals from random noise — and he hopes others will use it. But for now, the message is simple: Be sceptical of stock market strategies that rely on the calendar, the clouds — or the New York Mets.
"I mean, come on," Smith says. "Let’s be serious."
Latest news
- Why wealthy Americans work
An ASU economist's research shows that the affluent don't work for more stuff, but for better…
- ’Big league’ or big illusion? Study calls time on splashy stock market anomalies
In his latest research, an ASU professor invents a stock market anomaly to expose the shaky…
- What are the odds of a US recession? Here's how this economic expert calls it
There’s an epic level of uncertainty with the economy due to tariffs, said former U.S.